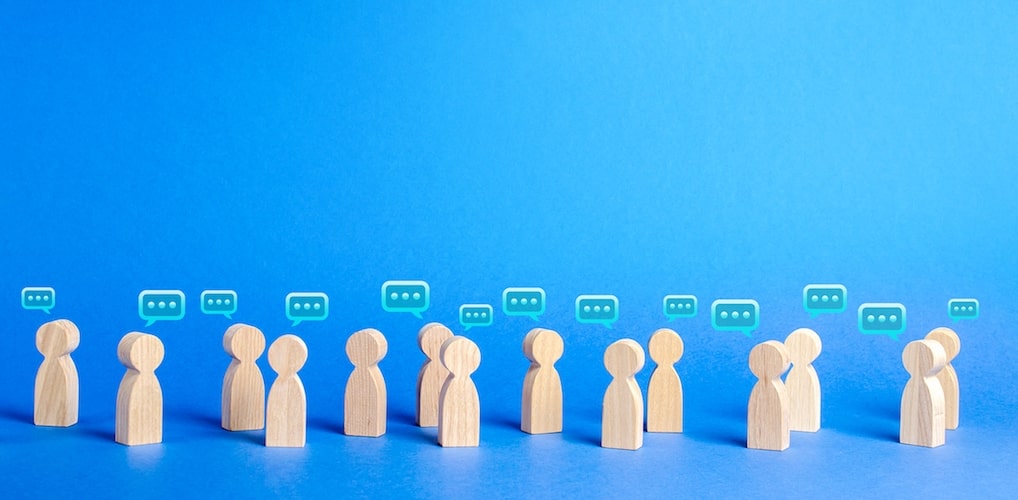
BlueML is Explorance’s new, purpose-built comment analysis solution, uniquely capable of providing rapid and in-depth insights into open text comments written by employees or students.
With BlueML, for the first time, the sentiment and experience locked away in surveys, websites, and social media can be reliably and rapidly processed – allowing organizations to better support the ongoing Employee and Student Experience.
The release of BlueML is the culmination of a substantial in-house development process led by Explorers Alexis Tremblay and Chanh T. Do.
In this blog post, we take a closer look at why and how BlueML was created, and the benefits for users.
Q. Could you give us a sense of the motivation and initial process that led to the development of BlueML?
Alexis: Sure. The initial starting point would likely be our existing provision of Text Analytics via Blue, our Experience Management (XM) platform. This was and is offered via a third-party tool, and we decided that it would be an improvement to transition to a Machine Learning (ML)-powered version of this tool.
This new solution would need to be designed so it could take advantage of the information the ML API was returning, which also gave us the opportunity to deliver further improvements, ranging from widgets to more granular demographics.
The work on developing what would become BlueML began in-house began in early 2019. What we’ve achieved has been the result of an in-depth collaboration between Explorance’s Machine Learning team and the Innovation Labs team.
Q. What is your elevator pitch for what BlueML delivers?
Alexis: It’s about finding out what people are really talking about and how they’re feeling – and being able to rapidly deal with the massively varied nature of these topics. Ultimately, it provides better and more actionable insights.
Sentiment analysis is certainly part of it, but it’s also about being able to process the hierarchical structure of topics. To use an example, we don’t just process furniture, we process varied combinations of chairs, tables, futons and so on. At the end of that process, a polarity is delivered, or a recommendation born out of that feedback is realized.
This is beyond simple text classification, which will tell you that Topic A and Topic B are being discussed. BlueML can do this, but also tells you that Topic A and Topic B are being discussed together.
We can attach a sentiment to every topic, so for a more client-specific example, instead of telling you about an instructor, and separately about grading, BlueML can tell you about the grading in relation to a particular instructor – but tell you if the comment is positive towards the instructor, but negative towards the grading. It allows for that kind of finer grain analysis.
This is core to the point – if you have text classification tools that don’t produce anything actionable, what can you really do with that? This is what differentiates BlueML.
Chanh: Additionally, BlueML continually adds more on top of (traditional text analytics). Text comments can be uploaded, and then an analysis can be made, which then can be utilized in a dashboard.
Let’s say for example, that the performance of a set of instructors needs to be assessed, as they’ve been receiving negative reviews. With BlueML, the nature of ongoing comments, and whether instructor ratings are improving or not, can be gauged.
This allows for both ongoing and retrospective insights, as more and more content is analyzed over a timeline.
Q. You mentioned that BlueML is the result of collaboration between the two teams. How would you describe the development and collaboration process between these two teams in Explorance?
Alexis: I would compare it to the development of a Formula 1 car. It’s a bit like the Machine Learning team has focused on building the engine that provides the power, and the Innovation Labs team has built and structured the car that surrounds and works off that engine. We’ve worked very closely to deliver this product.
All the computational power comes from the engine, while the aerodynamics that influence the motion of the car comes from BlueML – the applied capabilities of the solution itself.
The minute changes we would make to the car’s exterior might be our decision to track demographics, or to facilitate long-term tracking – which BlueML can do. This is all part of the fine-tuning that we’ve carried out with the solution that will greatly benefit users in the end.
Q. Was there any key development moment for the teams, when things took a step forward from that initial modest goal of improving the existing text analytics solution to something greater?
Alexis: Yes. While work began on the project in early 2019, very quickly, we recognized a new pathway that could deliver a more improved solution. This was the maturation of the process as new use cases emerged.
Our initial focus was on developing the text analytics solution in relation to Higher Education, we focused on this for about a year, as Explorance delivers solutions to assist Higher Education institutions and Enterprise-level businesses.
What we decided to do was apply what we had learned from that to Employee Learning Categorisation (ELC) and , which is the more corporate side of text analysis – and with a focus more purely related to the employee experience. We were able to transfer over what we learned from the education side to the corporate side.
The product has kept evolving, and now after two years in the making, we have the product we have today. This is a solution that, given the right data, can perform text analytics or comment analysis solution for any enterprise or business, or be used in higher education for feedback from students or employees.
Q. Was the development of the Machine Learning engine a first for Explorance? And does BlueML represent a first in the world of analysing comments using machine learning?
Alexis: This was a first for Explorance but it was a first beyond the company too. It was a brand new approach. We have not seen anything comparable to BlueML either in the literature on the topic, or anything produced (on the market).
For context: text analytics have been around for some time, and Machine Learning has long-since been employed to try to classify text. Generally, you would apply it to a block of text, and it could tell you, “Oh, this is talking about sports, about world politics, and other topics.” So that’s a multi-class definition. There could be 20 things being recognized as being talking about, but these are classed as being discussed as separate topics.
The next step is to multi-label. Maybe this block of text is talking about sports and politics at the same time. So, that can be applied too.
The next step is predicting the combinations of these labels together.
To put this in a workplace example, there could be a topic of communication, but it could be labelled as communication from the organization hierarchy as a whole, or as communication as carried out by your line manager. Communication is the same word, a topic and a workplace concern, but it all depends on what you relate it to, and how. In a nutshell, this is what we have been building with BlueML, a way of reliably recognizing this.
This, as far as we can tell, has not been done previously, and it requires a huge amount of data and a novel technique to deliver it. However, recent machine learning techniques such as neural networks and deep learning help to enable this approach. We lacked benchmarks to compare against, so we are very much creating our own baseline and expectations. It was uncharted territory.
Q. How much work had to be put into making the capability fit into an easy-to-use package, that works so well alongside the existing Blue XM platform for example?
Chanh: A fair amount of work was put into making the structure around the models, which allow users to upload their comments and receive predictions in return. There was also work put into classifying predictions. We also support the slicing and dicing of data after the analysis process. To break it down, what we made with BlueML was the support that a user could turn to, to explore predictions made after the analysis process.
We have a lot of experience in Explorance already working with data, it’s something Explorers already do, but this was not quite business as usual. Nevertheless, this product now tracks a very broad potential range of demographics, and that can be quite challenging to set up. We were able to successfully leverage our internal experience to realize this.
Q. What has been the initial feedback from the clients who have been able to try out BlueML?
Chanh: Largely, people are surprised in terms of the levels of analysis that are offered.
Alexis: What I’ve been hearing is that people are very impressed by the precision of the system, and how it’s performing in terms of its predictive insights. This was part of the initial design, we tried to prioritize precision over recall. This was a design decision that was made with an understanding that we may ultimately predict a smaller number of things, but that the precision will be higher.
When I talk about precision, I mean people scrutinizing information and considering “Is this right? Is this correct?” There is a consideration that the information may be missing something else, and that would be what we can “recall”. But typically, our judgement is that people will judge the system by the predictions they are provided, not the predictions that may be missing..
[“source=explorance”]